AGL is honing the machinery and processes it set up last year to smooth the path for machine learning models from proof-of-concept to production, with the process cut from 200 hours down to 20 hours.
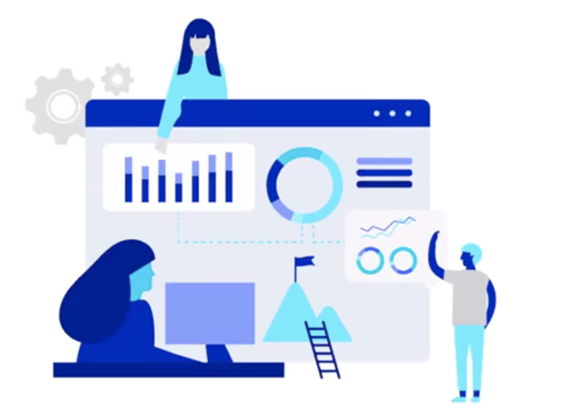
Head of advanced analytics Sarah Dods told IQPC’s Data Analytics online event series that the company used a ‘barn raising’ technique to stand up all the pieces needed for a production-use machine learning model.
“To actually do the technical work of bringing the model across [to pre-prod and then production], we’ve gone right back to the Amish and gone with ‘barn raising’ principles,” Dods said.
“In the Agile world, this is called swarming, but there’s some connotations around swarming and bees and we decided that ‘barn raising’ was a more positive term for the business to get their heads around.”
Barn raising is used to describe a process whereby machine learning engineers own specialist functions - such as governance and security, infrastructure support, release and deployment, data pipelines, and quality assurance - but work “in concert” to stand up the model in production.
By doing this a number of times, Dods said AGL had been able to create some reusable patterns to aid model transition, and in doing so had reduced the amount of hours per transition.
“Our first go at a transition from dev to pre-prod took 200 hours of effort,” she said.
“We’ve now got that down to 20 hours of effort, so it’s definitely getting more efficient as we go.”
AGL last year revealed it had centralised data analytics as an internal capability and set up a platform, mostly using Azure cloud services, to run “ML at scale”.
“This [platform] has been designed for us to be able to do both of the model development in the experimental stage and the productionisation and hosting [of] production [models] all within a single platform that’s designed to have … controls built in,” Dods said.
“It’s using a lot of the existing Azure functionality and [where] we found Azure didn’t have some of the functionality we needed to do this, Microsoft is actually extending the platform capabilities.
“They’re figuring if AGL has a challenge with it, then there will be other organisations that need to do the same thing.”
Despite the project effort and supporting platforms, Dods said the process of moving machine learning models from experiment to production use was still “quite hard”.
“Some of the things we’ve run into at AGL is that there’s not a good understanding of the investment that’s required to move a model from proof-of-concept to production,” she said.
“The work that’s been done may have been with a data extract rather than with data that’s sitting in an enterprise data system, so there’s some understanding that we need to get our upstream data pipelines in place.
“But we’re actually building a solution - not an algorithm - at this stage of the machine learning investment, and that means that there will be a downstream consumption system which will have its own software development lifecycle that we’re going to need to fit into for the data to be consumed.
“Somebody’s job has to change, and there has to be a system that’s going to present and manage and track that change.”
Dods noted there were also some fundamental differences between experimental and production machine learning models.
“When we’re looking at the proof-of-concept or experimental stage, what we’re generally trying to do is optimise on the mathematical or the technical side - how well can we make this model perform - and we’ll generally try out a bunch of different approaches and optimise across a number of techniques to get to that answer,” she said.
“That code has been written to explore many things rapidly at minimum cost.
“When we’re going up into the cloud and we’re looking at a solution that’s going to be delivered at scale, you need to do the re-coding to take advantage of all of the cloud options there around elasticity and around robust production-grade [services].”
With AGL moving machine learning capabilities to a central resource, there were also some cost of ownership challenges to consider.
“What we found is because we’re moving from a function that’s been distributed in the business, that sat within a business budget, to now something that sits within a technology function, there’s now centralised resourcing and costs to support and manage those models,” Dods said.
“[Those costs are] not expected by the business, because the people within their own business just used to take care of them.”
More models reach production
Still, AGL has used the new platform to bring several production use cases to life.
One of the more complex ones involves 4500 models that, together, are used for site-level forecasting down to an individual smart meter level.
“We have some large scale use cases that are really only possible with [our platform] and the automation we’ve got built into the system,” Dods said.
“Two of these are [for] site-level forecasts, so this is a many-models solution that predicts energy demands down to individual smart meter levels. It looks at consumption by the household, it looks at production via solar, and what gets used by a battery and what goes back into the grid. That work’s been done as part of our Virtual Power Plant development.
“It’s also used to plan energy trading responses to grid demand. For that we have 4500 models that get called every five minutes and get retrained every day.
“You can’t do that with manual retraining and manual monitoring. You need to have these enterprise-grade systems to be able to work at that level.”
Another production machine learning use case looks at stock forecasting of consumables used within AGL.
“Like any large corporates we buy a whole lot of things and we use them in different ways at different speeds in different places and at different times,” Dods said.
“We have a predictive model for each of those stock items or types of material, and those models are used to anticipate consumption and to optimise our ordering process.
“Those get retrained on a regular basis and, as you can imagine in a Covid-intense world, some of those consumption patterns are changing quite dramatically.
“We’re able to retrain automatically and keep those models current.”
Dods also briefly described a third machine learning model in production, “which is called the propensity to complain model, but is actually about improving customer service by understanding the issues that customers call us with, and how well we resolve them”, though she did not elaborate further.